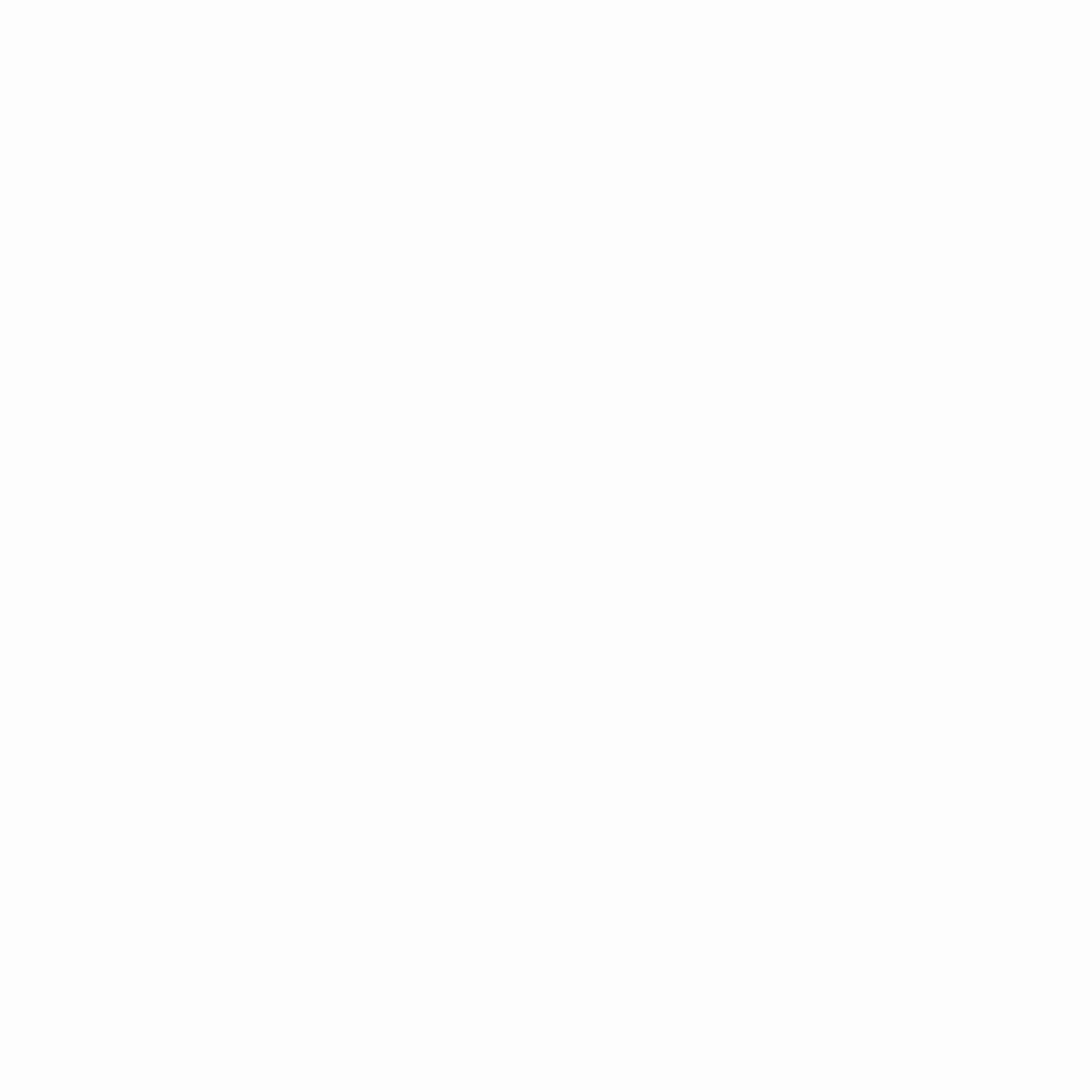
Conversational AI: Definition, Scope, Evolution, Applications and Features Explained
Conversational AI represents a revolutionary technology that enables machines to interact with humans through natural language processing and machine learning. This technology powers virtual assistants, chatbots, and other interactive platforms, allowing for seamless communication that feels increasingly human-like. As this field evolves, it promises to redefine how we interact with digital systems in our daily lives and businesses.
Key Takeaways
- Conversational AI leverages advanced technologies like NLP and machine learning to facilitate natural interactions between humans and machines.
- It has evolved from simple command-based systems to complex models capable of understanding context, intent, and nuances in conversation.
- Applications of conversational AI are widespread, enhancing customer service, streamlining operations, and providing personalized experiences.
- Building an effective conversational AI system involves careful planning, robust design, and continual optimization to meet user expectations.
The future of conversational AI includes further integration into daily life, ethical considerations, and continuous technological advancements.
Understanding Conversational AI
Definition and Scope
Conversational AI refers to the advanced technology that enables machines to engage in human-like dialogues. It harmoniously blends innovations in natural language processing, machine learning, and dialogue management. This technology allows computers to understand and respond to user inputs as if conversing with another human.
According to IBM, “Conversational artificial intelligence (AI) refers to technologies, such as chatbots or virtual agents, that users can talk to. They use large volumes of data, machine learning and natural language processing to help imitate human interactions, recognizing speech and text inputs and translating their meanings across various languages.”
Evolution and Technological Advances
The evolution of conversational AI has been marked by significant technological advances. From simple scripted responses to complex AI-driven interactions, the field has seen rapid growth. Key developments include enhanced voice recognition and adaptive learning algorithms that improve with each interaction.
Components of conversational AI
Conversational AI has principle components that allow it to process, understand and generate responses in a natural way.
Machine Learning (ML) is a sub-field of artificial intelligence, made up of a set of algorithms, features, and data sets that continuously improve themselves with experience. As the input grows, the AI platform machine gets better at recognizing patterns and uses it to make predictions.
Natural language processing is the current method of analyzing language with the help of machine learning used in conversational AI. Before machine learning, the evolution of language processing methodologies went from linguistics to computational linguistics to statistical natural language processing. In the future, deep learning will advance the natural language processing capabilities of conversational AI even further.
NLP consists of four steps: Input generation, input analysis, output generation, and reinforcement learning. Unstructured data is transformed into a format that can be read by a computer, which is then analyzed to generate an appropriate response. Underlying ML algorithms improve response quality over time as it learns.
These four NLP steps can be broken down further below:
- Input generation: Users provide input through a website or an app; the format of the input can either be voice or text.
- Input analysis: If the input is text-based, the conversational AI solution app will use natural language understanding (NLU) to decipher the meaning of the input and derive its intention. However, if the input is speech-based, it’ll leverage a combination of automatic speech recognition (ASR) and NLU to analyze the data.
- Dialogue management: During this stage, Natural Language Generation (NLG), a component of NLP, formulates a response.
- Reinforcement learning: Finally, machine learning algorithms refine responses over time to ensure accuracy.
How conversational AI works
According to Google, Conversational AI works by using a combination of natural language processing (NLP), foundation models, and machine learning (ML).
Conversational AI systems are trained on large amounts of data, such as text and speech. This data is used to teach the system how to understand and process human language. The system then uses this knowledge to interact with humans in a natural way. It’s constantly learning from its interactions and improving its response quality over time.
Benefits of conversational AI
1. Reduce costs, and increase productivity and operational efficiency through automation.
For example, conversational AI can automate tasks that are currently performed by humans and thereby reduce human errors and cut costs.
2. Deliver better customer experience, and achieve higher customer engagement and satisfaction.
For example, conversational AI can provide a more personalized and engaging experience by remembering customer preferences and helping customers 24/7 when no human agents are around.
Applications in Daily Life
Conversational AI has seamlessly integrated into daily life, enhancing user experiences across various platforms. Examples include:
- Virtual assistants on smartphones
- Customer service chatbots
- Voice-activated home assistants
- Interactive voice response systems in call centers
Building Blocks of Conversational AI
Natural Language Processing (NLP)
Natural Language Processing, or NLP, is the foundational technology that enables machines to understand and interpret human language. By analyzing the structure and meaning of words and sentences, NLP allows conversational AI to interact with users in a way that feels natural and intuitive.
Machine Learning and AI Models
Machine Learning and AI models are crucial for enabling conversational AI systems to learn from interactions and improve over time. These models analyze vast amounts of data to understand patterns and predict user responses, making the AI smarter with each conversation.
Dialogue Management Systems
Dialogue Management Systems orchestrate the flow of conversation. They determine the appropriate responses based on the user’s input and the context of the conversation. This system ensures that the conversation remains coherent and contextually relevant throughout the interaction.
Examples of conversational AI
Google has outlined some examples of conversational AI. A few application examples of conversational AI technology:
- Generative AI agents: these virtual agents use generative AI to power text or voice conversations.
- Chatbots: often used in customer service applications to answer questions and provide support.
- Virtual assistants: often voice-activated and can be used on mobile devices and smart speakers.
- Text-to-speech software: used to create audiobooks, or spoken directions.
- Speech recognition software: used to transcribe lectures, phone calls, captions, and more
Designing a Conversational AI System
Planning and Design
Designing for conversational AI is the process of creating interactions that feel natural and intuitive. This phase involves defining the purpose, scope, and functionalities of the AI system. Key considerations include user needs assessment, persona development, and conversation flow mapping.
Development and Integration
Once the design is finalized, the development phase involves building the conversational AI using appropriate technologies. Integration with existing systems and databases is crucial for seamless functionality. This stage often requires iterative adjustments and enhancements based on user feedback.
Testing and Optimization
The final stage focuses on ensuring the conversational AI performs as intended. Rigorous testing for understanding accuracy, response time, and user satisfaction is essential. Optimization efforts might include refining NLP capabilities and expanding the system’s ability to handle varied user inputs.
Conversational AI in Business
Enhancing Customer Service
Conversational AI significantly improves customer service by providing real-time support and personalized interactions. Businesses can automate responses and handle multiple customer queries simultaneously, leading to increased satisfaction and loyalty.
Streamlining Operations
Conversational AI plays a crucial role in optimizing business operations. It automates routine tasks, such as scheduling and data entry, freeing up human resources for more complex issues. This efficiency not only saves time but also reduces operational costs.
Gathering Insights and Analytics
Conversational AI is instrumental in collecting and analyzing vast amounts of data. This capability allows businesses to gain deep insights into customer behavior and preferences, which can be used to refine marketing strategies and enhance user experiences. The analysis of user sentiments helps in continuously improving the services offered. Read More
Challenges in Conversational AI
Understanding Context and Nuance
Conversational AI often struggles with understanding context and nuance, which can lead to misinterpretations and unsatisfactory user interactions. This challenge is particularly pronounced in complex conversation scenarios where multiple topics or subtle cues are involved.
Handling Multiple Languages
Handling multiple languages effectively remains a significant hurdle for conversational AI. Each language has its own set of rules, idioms, and cultural nuances that AI must navigate. This diversity requires sophisticated models that can adapt to various linguistic structures and meanings.
Privacy and Security Concerns
Privacy and security are paramount in the deployment of conversational AI systems. Users’ sensitive information must be protected from breaches and misuse. Ensuring robust security measures and maintaining user trust are critical for the widespread adoption of conversational AI technologies.
The Future of Conversational AI
Trends and Predictions
The landscape of conversational AI is rapidly evolving, with multi-bot experiences and metaverse integration leading the charge. Enhanced contextual awareness will allow these systems to provide more nuanced and effective interactions. The integration of AI across different platforms and devices is expected to become more seamless, enhancing user experience significantly.
Potential for Growth
Conversational AI is poised for substantial growth in various sectors including healthcare, finance, and customer service. The technology’s ability to scale personalized interactions without compromising quality makes it a valuable asset for businesses looking to enhance their service offerings.
Ethical Considerations
As conversational AI technologies advance, ethical considerations become increasingly important. Ensuring that these systems are designed with fairness, transparency, and respect for user privacy will be crucial. It is essential to address these ethical challenges proactively to build trust and ensure the responsible deployment of AI technologies.
Conclusion
Conversational AI represents a significant leap forward in how humans interact with technology. By integrating advanced machine learning, natural language processing, and dialogue management, these systems offer a seamless, intuitive communication experience that mimics human conversation. As we’ve explored in this article, the applications of conversational AI are vast, from enhancing customer service to supporting personal assistants and beyond. The continuous advancements in this field promise even more sophisticated interactions in the future, potentially transforming our daily interactions with machines into more natural and engaging exchanges.
Frequently Asked Questions
WHAT IS CONVERSATIONAL AI?
Conversational AI refers to the cutting-edge field that involves creating computer systems with the ability to engage in human-like and interactive conversations. It harmoniously blends innovations in the field of natural language processing, machine learning, and dialogue management to achieve highly intelligent bots for text and voice channels.
How do you build a Conversational AI?
Building a conversational AI involves integrating several components such as natural language processing (NLP), machine learning models, and dialogue management systems. The process includes planning and design, development and integration, followed by testing and optimization to ensure smooth and intelligent interactions.
How can a Conversational AI platform help?
A conversational AI platform can enhance customer service, streamline operations, and gather valuable insights and analytics. It provides a more immersive and engaging user experience by interpreting context, understanding user intents, and generating intelligent, contextually relevant responses.
What is NLP in Conversational AI?
NLP, or Natural Language Processing, is a crucial component of conversational AI. It refers to the technology that enables computers to understand, interpret, and generate human language in a way that is both meaningful and useful. In conversational AI, NLP is used to process and analyze human language data, allowing systems like chatbots or virtual assistants to engage in dialogue with users in a natural and intuitive way. This involves various tasks such as speech recognition, language understanding, and language generation, enabling these systems to handle queries, provide responses, and sustain a conversation.
What is the difference between conversational AI and generative AI?
Conversational AI is focused on creating interactions that mimic human conversations, using technologies like NLP and machine learning to understand and respond to inputs. Generative AI, on the other hand, extends beyond conversation to generate new content, ideas, or data based on trained models.
What are the challenges in developing Conversational AI?
Developing conversational AI faces challenges like understanding context and nuance, handling multiple languages, and ensuring privacy and security. These challenges require sophisticated AI models and continuous optimization to ensure accurate and secure user interactions.
copyright@aireporter.news