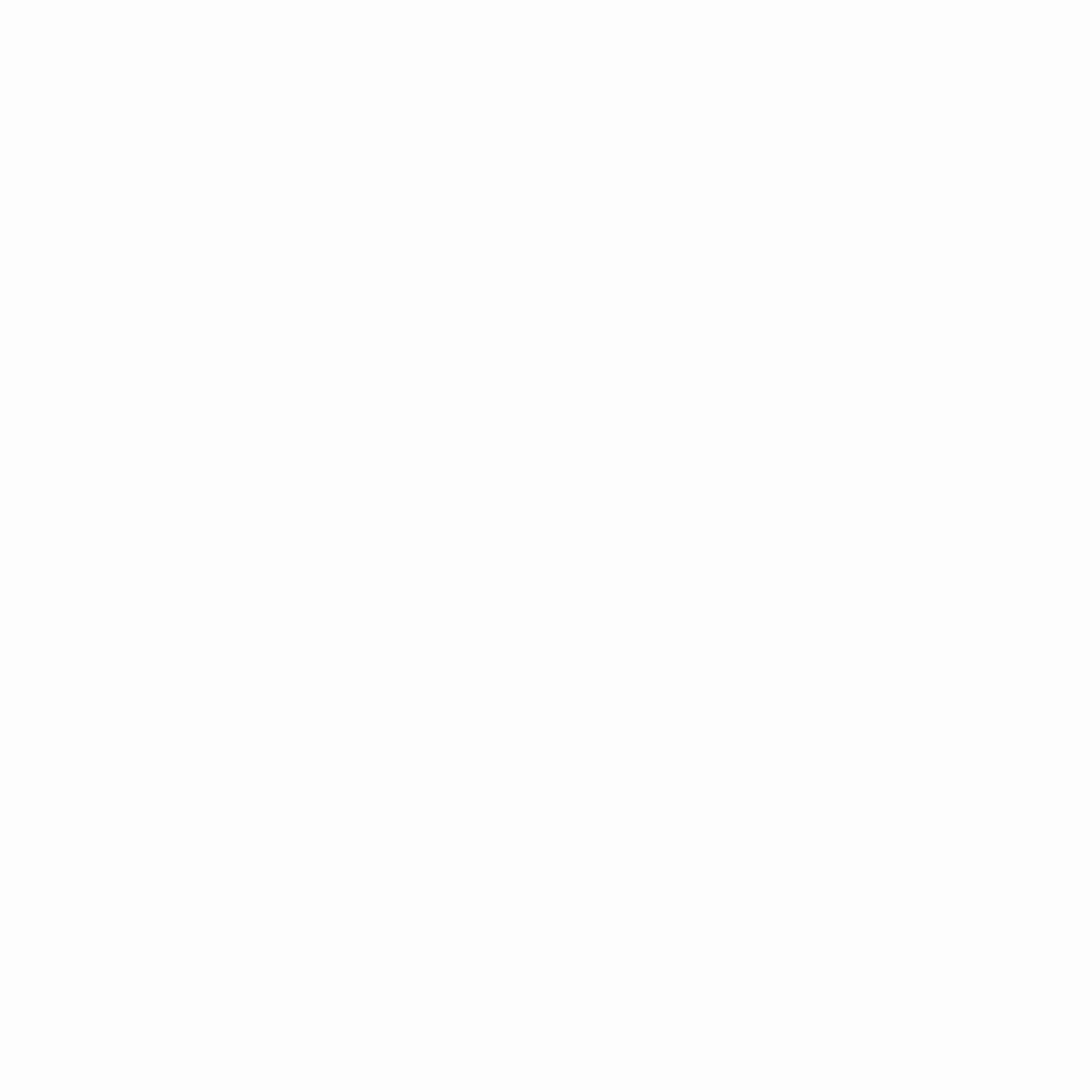
Central Banks Use Artificial Intelligence (AI) to Tackle Climate Risk Assessment
New Tool Streamlines Data Collection from Company Disclosures
LONDON (AI Reporter/News): In a significant development, central banks have announced a breakthrough in utilizing artificial intelligence (AI) to analyze climate-related financial risks. This innovation comes at a crucial time as the volume of climate disclosures from companies is poised to surge.
The Bank for International Settlements (BIS), a central bank forum, collaborated with the Bank of Spain, Germany’s Bundesbank, and the European Central Bank on the experimental project, codenamed “Gaia AI.”
The Bank for International Settlements (BIS): A Global Hub for Central Banks
Founded in 1930, the Bank for International Settlements (BIS) is an exclusive club for central banks. With 63 members representing a whopping 95% of global GDP, the BIS fosters international cooperation on financial matters. Headquartered in Basel, Switzerland, it has a global presence with representative offices in Hong Kong and Mexico City, along with Innovation Hub Centers scattered around the world.
This project focused on extracting and analyzing data from company disclosures concerning carbon emissions, green bond issuance, and voluntary net-zero pledges.
Project Gaia has built a new AI-based application to better assess climate-related risks to the financial system.
Bank for International Settlements
Project Gaia aims to help analysts search corporate climate-related disclosures and extract data quickly and efficiently using artificial intelligence (AI), particularly large language models (LLMs), the report revealed.
“Gaia Phase I has surveyed climate risk experts from central banks and supervisory authorities, designed a solution that addresses the requirements articulated by these experts and delivered a proof of concept (PoC) demonstrating the technical feasibility of the concept.”, the report said.
Challenges of Fragmented Data Hamper Assessment
Financial regulators overseeing banks, insurers, and asset managers require high-quality data to accurately assess the impact of climate change on financial institutions. However, the lack of a universal reporting standard creates a major hurdle. Public information currently exists in a fragmented format, scattered across text, tables, and footnotes within annual reports.
Gaia: A Beacon of Transparency in a Sea of Disparity
The central banks, in a joint statement, highlighted Gaia’s ability to surpass discrepancies in definitions and disclosure frameworks across different jurisdictions. This paves the way for much-needed transparency and facilitates comparisons of climate-related financial risk indicators.
“Generative AI promises to change the way we work in the future, and Project Gaia is one of the first comprehensive studies investigating how this can be done in practice.”, the report said.
***
AI Cuts Through Labeling Variations to Extract Meaning
Despite inconsistencies in how companies report the same data, Gaia prioritizes the underlying definition of each indicator, instead of getting bogged down by labeling variations. Traditionally, analyzing additional key performance indicators (KPIs) or incorporating new institutions involved manual searches through company reports or direct contact with the institutions – a time-consuming and laborious process.
“Gaia is a game-changer,” stated a representative from the central banks. “Adding new KPIs or institutions is now effortless. This unlocks the potential for extracting and analyzing a vast array of KPIs from a multitude of institutions, enabling climate risk analysis on a previously unimaginable scale.”
Project Gaia: Automating Workflows for Powerful Financial Analysis
Project Gaia exemplifies the power of AI-enabled tools to streamline existing processes. The report highlights macro analysis results covering a massive dataset: 20 key performance indicators (KPIs) for 187 financial institutions over five years. And here’s the beauty: adding more institutions or KPIs is a breeze. This flexibility extends beyond climate-related data analysis, showcasing the broader potential of AI-powered applications for central banks and the entire financial sector.
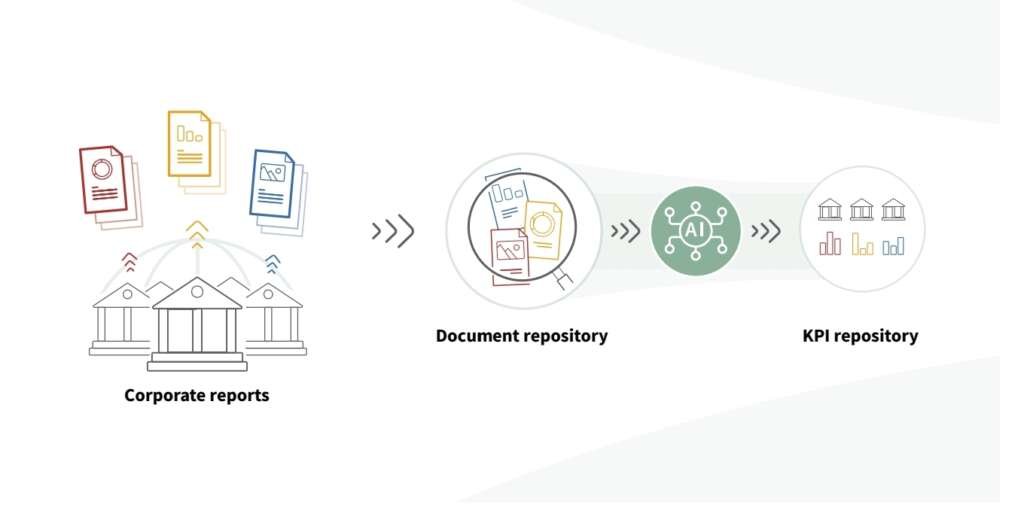
Mandatory Disclosures Ushering in the Era of Detailed Data
Listed companies, including banks and insurers, are facing new mandatory climate-related disclosure requirements under recently implemented global, U.S., and European Union regulations.
This shift represents a significant step forward from the voluntary approaches used previously, promising a more comprehensive information landscape.
Initial Findings: Uneven Progress on Green Commitments
Gaia’s analysis of 20 key indicators for 187 financial institutions over a five-year period, based on documents in English, Spanish, and German, revealed a rise in the number of financial firms setting net-zero targets and issuing green bonds. However, the data also suggests a geographically uneven distribution of these commitments.
***
Data processing and storage
Data processing is performed by four data processing pipelines:
- The PDF parsing pipeline.
- The KPI extractor pipeline.
- The open query pipeline.
- The structured data exploration pipeline.
The pipelines and the data storage components are illustrated in Graph. Raw input files – a library of corporate reports – reside in the document repository, which is implemented in Azure Data Lake.
The PDF parsing pipeline takes its input from the document repository in the form of PDF documents. The documents are processed using the Azure AI Document Intelligence into a machine-processing-friendly JSON format and stored alongside the original documents.
Next, the documents are split into individual pages and uploaded into the page repository, an OpenSearch document database. For each page, a vector embedding is calculated using sentence transformer models and this is stored in the metadata of the respective page record.
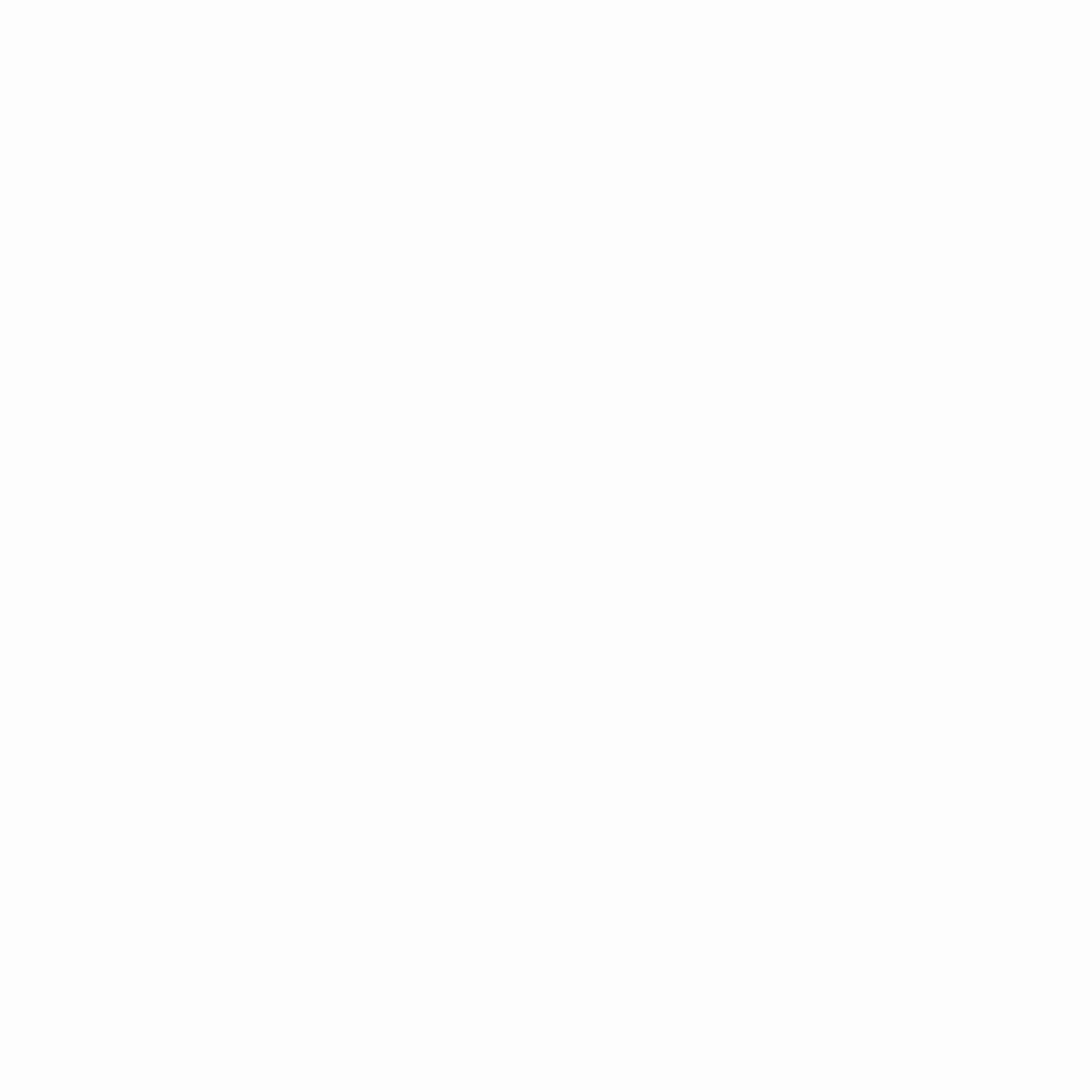
Gaia consists of a set of automated processing pipelines, transferring newly submitted reports to the page repository and extracting KPI into the KPI repository. From this pipeline, specific use cases are implemented to serve Gaia’s customers.
***
Beyond Climate Risk: A Broader AI Vision
“Gaia’s flexible design has the potential to serve as a model for AI-enabled applications across a wider range of use cases for central banks and the financial sector,” the central banks remarked.
They also revealed that making Gaia publicly available as an open web-based service for analysts is a potential next step.
This pioneering use of AI by central banks signifies a critical step forward in effectively assessing climate-related risks within the financial system. With the potential for broader applications and the promise of open access, Gaia’s impact could extend far beyond the realm of climate risk analysis.
***
Climate Data Conundrum: Challenges Hinder Financial Institutions’ Risk Assessment
Climate change poses a significant financial risk to institutions, demanding a thorough assessment of their exposure. This report explores the hurdles hindering this crucial analysis.
The report identifies two main categories of climate-related financial risks: physical risks stemming from extreme weather events and transition risks arising from the shift to a sustainable economy. Central banks and supervisors require high-quality, comparable, and accessible data to assess the financial system’s vulnerability to these risks.
While a company’s environmental, social, and governance (ESG) disclosures offer valuable insights into its climate practices, several challenges impede the availability of this information:
Standardization Woes: A lack of consistent global standards for ESG reporting leads to a confusing array of frameworks and metrics. This makes it difficult to compare ESG indicators across different companies and industries.
Data Quality Concerns: Ensuring the accuracy and reliability of ESG data is challenging as it often relies on self-reported information and proxies, which can be prone to errors, bias, and a lack of transparency.
Scope and Materiality Quandary: Determining which ESG factors are most significant to a company’s performance and which need to be disclosed is an ongoing struggle, as these factors vary by industry and context.
Regulatory Labyrinth: The patchwork of ESG reporting regulations across different regions creates a complex and fragmented landscape.
Manual Data Extraction Burden: Collecting climate-related indicators from corporate ESG reports is often a time-consuming manual process. The relevant information is scattered across various reports and may be presented in a combination of text, tables, footnotes, and figures, requiring extensive analysis to extract the desired data point.
***
Project Gaia Overview
Revolutionizing Climate Data Analysis with AI
Fact | Brief |
---|---|
Objective | AI-enhanced search and extraction of climate-related disclosures. |
Phase I | Designed solution and PoC based on climate risk expert feedback. |
Benefits | Automated extraction offers comprehensive analysis and harmonized metrics. |
Challenges | Overcame LLM issues like response times and hallucinations through design innovations. |
Application | Integrated LLMs for efficient climate disclosure data extraction. |
Design | Scalable platform covering 20 KPIs for 187 institutions over five years. |
Impact | Potential for broader use in financial sector and central banking. |
***
7 FAQs Regarding the Climate Data Challenge and its Impact on Financial Stability:
Q1. What are climate-related financial risks?
Climate-related financial risks refer to potential risks that may result from climate change and could potentially impact the safety and soundness of individual financial institutions and have broader financial stability implications for the banking system1. These risks are typically classified into two categories:
Physical Risks: These stem from the increased frequency of extreme weather events, rising temperature, and sea level rise. They can affect various sectors of the economy and may affect access to financial services.
Transition Risks: These result from rapid repricing of financial assets due to changes in policies or shifts in consumer and investment demand. Transitioning to a lower-carbon economy may entail extensive policy, legal, technology, and market changes to address mitigation and adaptation requirements related to climate change.
These risks are recognized by most banks as a material threat to their business5. The urgency of these issues has led to increased focus on climate and environment-related risks, and some banks are also focusing on the social and governance aspects while evaluating credit proposals.
Q2. How does climate change affect financial stability?
Climate change can significantly impact financial stability through various channels:
Asset Price Volatility: The manifestation of physical risks could lead to a sharp fall in asset prices and increase in uncertainty. This could alter asset price (co-)movement across sectors and jurisdictions.
Credit, Liquidity, and Counterparty Risks: Abrupt increases in risk premia across a wide range of assets could amplify these risks. This could challenge financial risk management in ways that are hard to predict.
Risk Diversification and Management: Changes in the financial system may weaken the effectiveness of some current approaches to risk diversification and management1. This may in turn affect financial system resilience and lead to a self-reinforcing reduction in bank lending and insurance provision.
Disorderly Transition Risks: A disorderly transition to a low carbon economy could also have a destabilising effect on the financial system. Financial market participants that do not put in place frameworks to assess and address climate-related risks could face significant losses on climate-sensitive assets caused by environmental shifts, by a disorderly transition, or both.
Data Gaps: The efficacy of actions taken by financial firms to reduce or manage their exposure to climate-related risks may be hampered by a lack of data with which to assess clients’ exposures to climate-related risks, or the magnitude of climate-related effects.
Q3. Why is ESG disclosure important for addressing climate risks?
ESG (Environmental, Social, Governance) disclosures are crucial for addressing climate risks due to the following reasons:
Transparency and Accountability: ESG disclosures provide insights into a company’s climate practices, fostering transparency and accountability in managing climate-related risks. This helps stakeholders, including investors and customers, understand how a company is addressing these risks.
Informed Decision Making: ESG disclosures provide reliable information about climate risks, enabling investors to make informed investment decisions. Without such disclosures, there could be poor investment decisions, asset losses, and continuation of trade practices that contribute to climate change.
Risk Management: ESG disclosures can help companies manage their climate risks more effectively. They provide a framework for companies to assess their exposure to climate risks and take appropriate mitigation measures.
Regulatory Compliance: There has been an increasing number of regulatory initiatives globally to accelerate the assessment of banks’ exposures to and management of climate risks, notably through stress tests. ESG disclosures can help companies comply with these regulatory requirements.
Reputation Management: Companies that fail to disclose their climate change risks could jeopardize their reputations1. ESG disclosures can help companies maintain their reputation by demonstrating their commitment to addressing climate risks.
Q4. What challenges are associated with ESG reporting?
While ESG reporting is essential for sustainable business practices, it comes with its own set of challenges. ESG (Environmental, Social, Governance) reporting, while crucial, faces several challenges:
Lack of Standardization: There are multiple ESG frameworks, such as the Global Reporting Initiative, the EU Taxonomy, the Sustainability Accounting Standards Board, and the Task Force on Climate-related Disclosures.
The absence of a single, global standard for ESG reporting leads to inconsistencies and makes it difficult for organizations to choose the most appropriate framework.
Data Quality and Accuracy: Ensuring the quality and accuracy of ESG data is a significant challenge. Companies often struggle with data availability, collection, and verification.
Determining Scope and Materiality: It can be challenging for companies to determine the scope of their ESG reporting and to identify the material aspects that should be included.
Regulatory Fragmentation: The regulatory landscape for ESG reporting is evolving and varies across regions1. This fragmentation can make it difficult for companies, especially those operating in multiple jurisdictions, to comply with all applicable regulations.
Complex ESG Data Management: Managing ESG data is complex due to the vast amount of information that needs to be collected, processed, and reported.
Q5. Why is it difficult to extract and compare ESG indicators?
Extracting and comparing ESG indicators across companies is indeed a complex task due to several reasons:
Multiple ESG Frameworks: There are numerous ESG frameworks, each with its own set of metrics. This variety makes it challenging to extract and compare ESG indicators across companies, as they might adhere to different frameworks.
Inconsistent Global Standards: The lack of a single, global standard for ESG reporting leads to inconsistencies in the data reported by different companies. This inconsistency hampers the extraction and comparison of ESG indicators.
Complex ESG Data Management: The vast amount of information that needs to be collected, processed, and reported for ESG makes data management complex1. This complexity can make it difficult to extract and compare ESG indicators.
Evolving ESG Regulations: The regulatory landscape for ESG reporting is continuously evolving and varies across regions. This constant change can complicate the extraction and comparison of ESG indicators.
Materiality Assessment: Companies need to identify the ESG issues that have a high impact on their business operations. This requirement can lead to differences in the KPIs that companies prioritize, further complicating the extraction and comparison of ESG indicators.
Q6. What complicates the collection of climate-related data from corporate reports?
Collecting climate-related data from corporate reports is a complex task due to several reasons:
Diverse Formats: Corporate reports present information in various formats such as text, tables, and figures. This diversity requires significant effort to understand and extract relevant data.
Dispersed Information: Climate-related data is often scattered across multiple sections or even multiple reports. Locating and compiling this data can be time-consuming and challenging.
Inconsistent Reporting Standards: There may be inconsistencies in how different corporations report their climate-related data. Some might provide detailed data, while others might only offer summaries.
Technical Language: The data in these reports is often presented in technical language specific to environmental science or corporate finance. This can make the data difficult to understand for those without expertise in these areas.
Time-Consuming Manual Effort: The process of sifting through these reports, understanding the context, extracting the relevant data, and then standardizing it for comparison or analysis requires significant manual effort.
Q7. How do divergences in ESG ratings affect the reliability of ESG data?
Divergences in ESG (Environmental, Social, and Governance) ratings can indeed affect the reliability of ESG data. Here’s how:
Different Interpretations: ESG rating agencies often have different methodologies for interpreting and scoring ESG data. This can lead to significant divergences in the ratings assigned to the same company by different agencies.
Subjectivity: The interpretation of ESG data can be subjective, depending on the weightage given to different factors. For instance, one agency might prioritize environmental factors more heavily, while another might focus more on governance.
Lack of Standardization: There is currently no universally accepted standard for ESG reporting. This means that companies often report different types of data, making it difficult to compare ratings across companies or industries.
Need for Granular Data: Divergences in ratings highlight the need for more granular, detailed data. More specific data can help reduce subjectivity and improve the accuracy of ESG ratings.
These factors can question the reliability of ESG data and make it challenging for investors and other stakeholders to make informed decisions based on these ratings. Therefore, efforts are being made to standardize ESG reporting and improve the transparency and comparability of ESG data.
copyright@aireporter.news