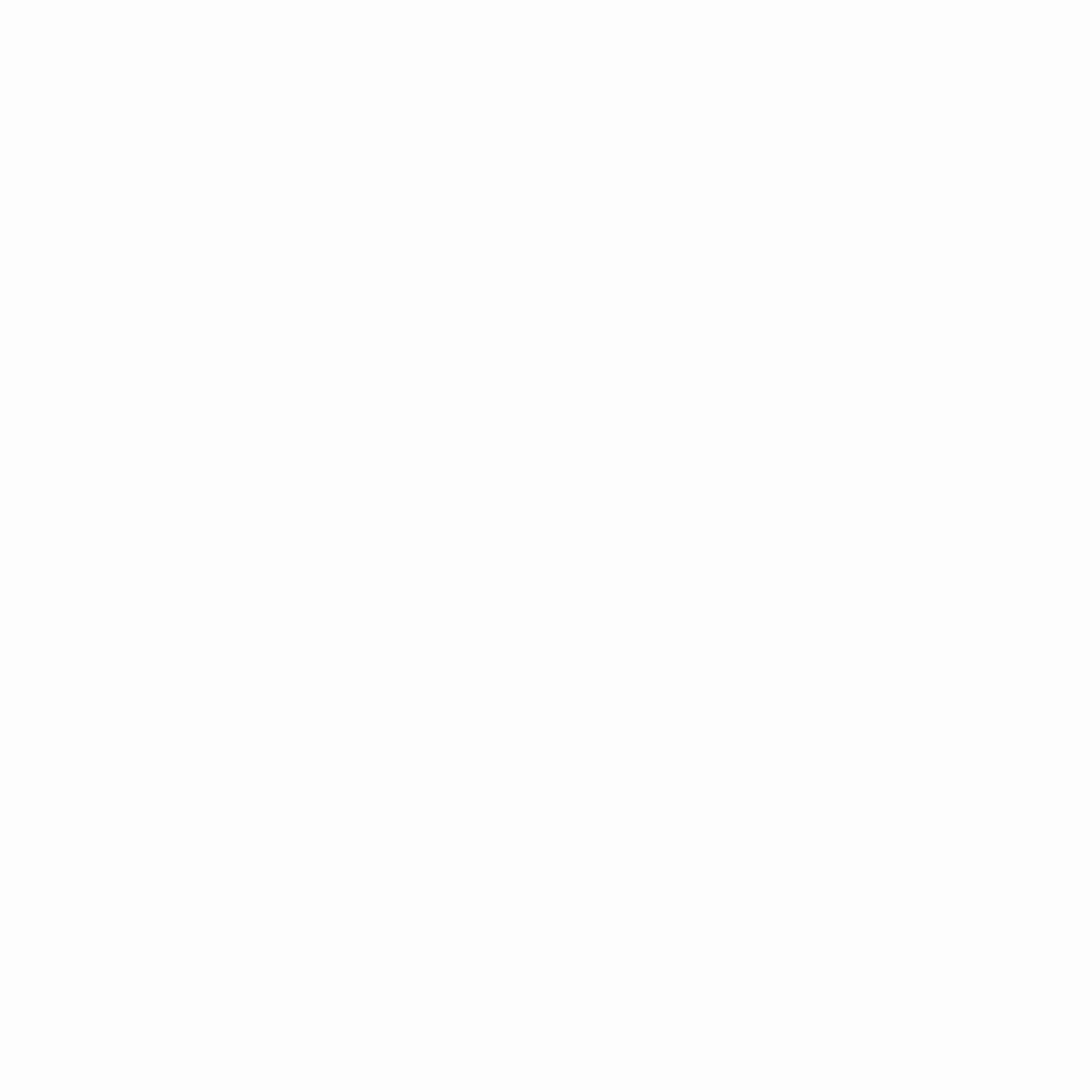
AI in Sustainable and Responsible Exploration of Rare Earth Elements
Learn how AI can transform rare earth exploration into sustainable, responsible practices for a greener future.
Words Nihar Ranjan Sahoo
AI in Sustainable and Responsible Exploration of Rare Earth Elements
In the context of net-zero targets, the global energy crisis, and the shift away from fossil fuels, Rare Earth Elements (REEs) are emerging as primary sources of renewable energy, complementing solar, wind, geothermal, and tidal energy. Lanthanides and yttrium, constituting REEs, have gained prominence due to their limited availability and high demand in various consumables and industrial applications, notably in advanced products like permanent magnets, batteries, catalysts, computer memory, and lighting.
The economic viability of extracting REEs, given their highly dispersed and low-concentration availability, is a significant challenge. Moreover, conventional extraction methods often contribute to environmental damage, compounded by competition for land use. Geologists are now exploring alternative sources, such as placer deposits and recycling, for optimized and precise exploration of these critical resources without undue environmental impact.
1. Use Cases and Global Scenario of REEs
Rare earth elements, a group of 17 metallic elements, are vital components in a myriad of high-tech consumer products and military applications. Their use has become increasingly important, with estimates suggesting they are essential in over 200 products. Applications range from consumer electronics, like phones and computer hard drives, to automotive components, particularly in electric and hybrid vehicles, where REEs are integral to efficient operation.
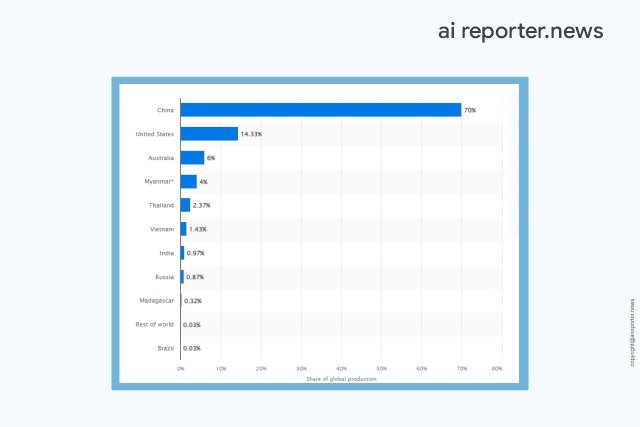
As of 2022, China dominated global rare earth mining production, accounting for over two-thirds of the total, while the US trailed as a distant second with a 14.33% share. Despite their relatively small fraction in weight, value, or volume, REEs are indispensable for the proper functioning of many everyday products, as well as the development of cutting-edge technology and defense systems. However, concerns persist about the sustainability and geopolitical implications of concentrated REE production in a few countries.
2. AI in Mineral Exploration
Mineral Exploration looks for diverse and disparate geosciences data to collate and synthesize data from varied primary and secondary sources including Satellite Imagery to help derive insights in reconnaissance study, mineral mapping, and evaluates alternate options in precision exploration.
Varied High Resolution Satellite imagery augmented with drone-based imagery along with mathematical models using varied AI/ML algorithms harnesses the power of geosciencesin recognition and utilization of complex patterns in the complex multi-dimensional data, enabling valuable inferences- delineate mineralization and their grade, and tonnage and other functionality.
Next steps are to mine with precision blasting and exploration of mineralization from overburden (gangue) with large operational efficiency and simultaneously complying to sustainability goals.
Mineral Exploration, AI and Open Data for Sustainability
The mineral exploration industry faces challenges in processing vast amounts of data from diverse sources, often without yielding significant discoveries.
With the advent of open data initiatives including Open Subsurface Data Universe (OSDU) and technologies like earth observation, which includes optical, radar, hyperspectral, gravity, aeromagnetic, and radiometric surveys, coupled with computer vision and predictive analytics, the creation of a spatial decision support system has become more accessible.
This aids in targeting and delineating prospective mineral resources, optimizing business processes, and supporting sustainable development goals.
AI-based mineral exploration algorithms
AI-based mineral exploration algorithms, along with drones for autonomous drilling, contribute to cost-effective exploration. Cloud-based image analysis, coupled with augmented analytics, efficiently categorizes and segments satellite imagery, providing geologists with valuable insights over large areas, enhancing discovery rates and expediting the exploration life cycle.
Recent literature talks of AI, particularly convolutional neural networks (CNN) and deep reinforcement learning, showing promise in precisely estimating REEs based on 3D mineral perspectivity from geological models.
Machine learning techniques, including fully connected neural networks (FCNN), are being employed to identify optimal ligands for separating trace elements stacked with REEs. Commercially, AI has been successfully used in stockpile management, stope volume estimation, pit optimizations, and more.
3. REE and the Sustainability Megatrend
As an integral part of digital infrastructure, REEs play a crucial role in high-tech products and renewable energy sources. The precise exploration of REEs using disruptive technologies like AI/ML, Industrial Internet of Things (IIOT), space technology, and the metaverse aligns with sustainability goals in mining. By leveraging these capabilities, businesses can move closer to a greener future and contribute to the global sustainability megatrend.
***
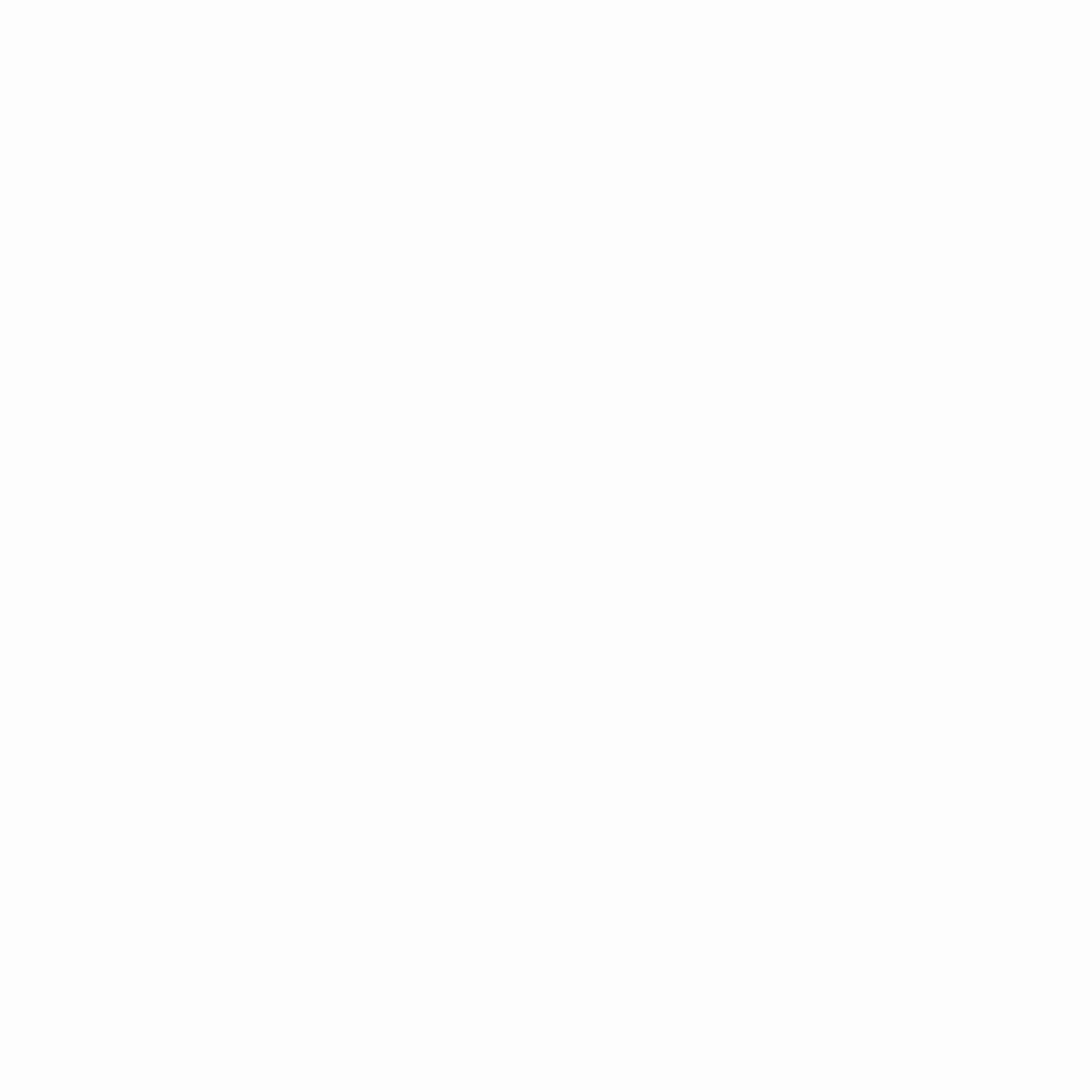
About the author
Nihar Ranjan Sahoo I Senior Principal Architect & Head – Geospatial Technologies. Nihar is a PhD in Earth Sciences with specializations in GIS, Remote Sensing, Applied Statistics and has over 23 years of industry experience. His interest areas lie in building end-to-end development, deployment and operationalization of Geospatial Machine Learning Solutions with Earth Observation Data.
Views are personal.
copyright@aireporter.news