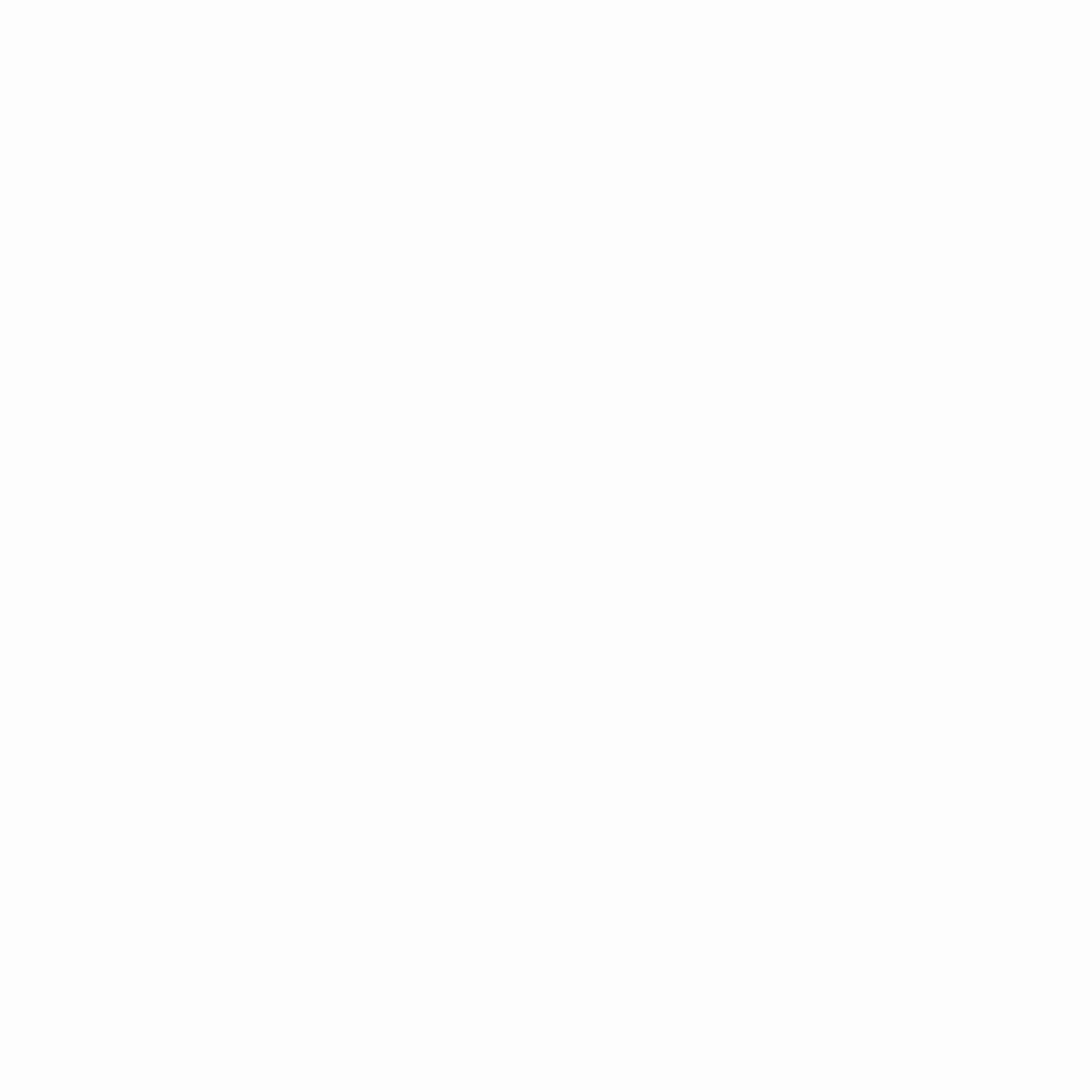
AI Makes Software Testing Irrelevant Or Does it?
The rapid advancement of technology has highlighted the significant role of AI in software testing, igniting a broad discussion. While there are concerns that AI might supplant human testers, many assert the irreplaceable value of human insight in guaranteeing the software’s readiness and effectiveness for users.
The article will explore key issues such as:
1. Will AI replace software testing?
2. Why AI won’t make Software Testing irrelevant
3. AI technology will create more jobs than it automates
Words Rajat Taheem
The goal of artificial intelligence (AI) in software testing is to make testing better and more effective. To automate and improve testing, AI and machine learning leverages reasoning and problem-solving, helps software testing teams focus on more complicated duties, such as building inventive new features, by
reducing on the need for time-consuming manual testing.
As technology continues to evolve at a rapid pace, the role of AI in testing has become a topic of great interest and debate. While some feel that AI will eventually replace human testers, others feel that the human element is important to ensure that the software is ready for a user and effective.
So, will AI replace software testing?
But here’s what we’ve been overlooking: Many new jobs will also be created – jobs that look nothing like those that exist today.
Let’s understand this by taking a real-world example.
***
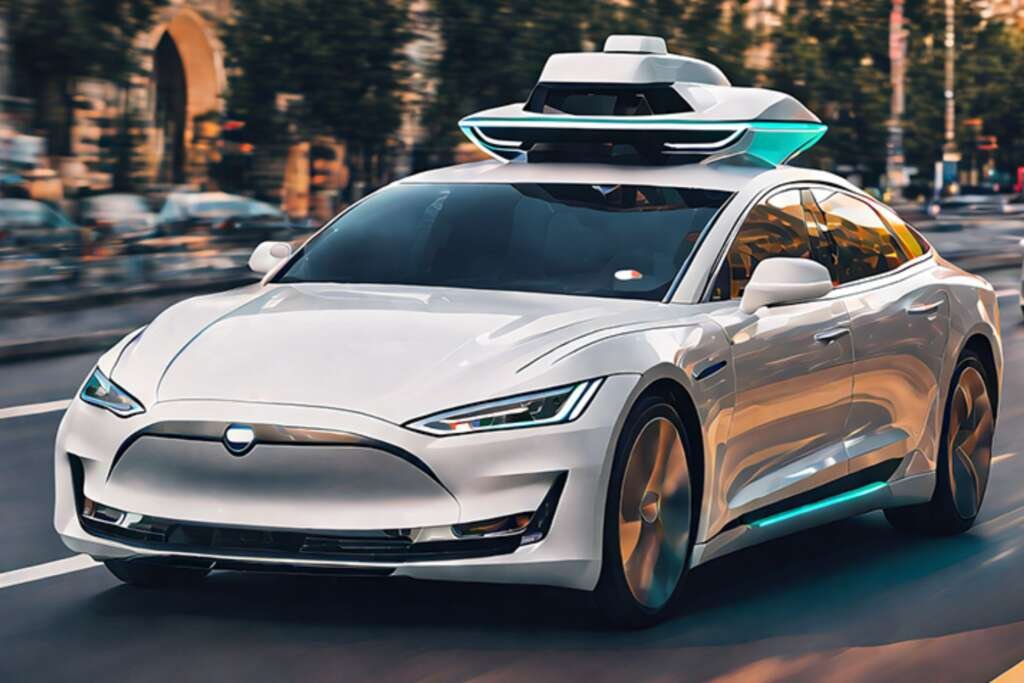
Autonomous Vehicle Industry
Self-driving cars need a considerable amount of testing to ensure that the vehicle is ready and safe for public use. This is where AI comes in. Leading self-drive vehicle manufacturers use machine learning algorithms to analyze immense amount of data gathered from their vehicles.
This data is then used to identify potential issues and optimize the performance of their self-driving cars.
However, while AI can be useful in this context, these companies still employ humans to conduct manual testing of their software. Humans engineers continue to be an essential part to add machine learning data for AI to test.
Let’s now look at another scenario:
Once upon time, there was a software development company that had been struggling to keep up with the demands of its clients. The company’s testing process was slow and laborious, often resulting in delays. As
a result, the company’s reputation had begun to suffer.
While looking for a solution, the company came across the idea of AI in testing and adopted it to automate the testing process and reduce the time required.
At first, the results were impressive. The AI software was able to quickly run through a large number of test cases, identifying bugs that human testers might have missed. This led to faster and efficient testing, and the company’s clients were impressed.
However, as time went on, the limitations of AI software began to become apparent. While software was able to identify many bugs, it was not always able to understand the context in which those bugs existed.
This led to false positives and false negatives.
Creating these AI bots to test application is again prepared by humans only. It is effort of all humans involved that evolve these AI capabilities.
Eventually the company adopted to a hybrid approach toward software testing, using both AI and human testers to identify bugs and defects. The AI software was used to automate repetitive tasks and identify hard to find bugs, while human testers were used to provide context and critical thinking skills that AI lacked.
All the lessons learnt was added as Machine Learning.
***
What This Means?
It is same boom as created by ERP earlier. Did ERP applications cut jobs? It actually created several new ones. Many feared that implementation of ERP would eventually lead to job loss or would actually scale down manpower.
Turns out, it actually added more jobs. All people had to do was adapt & reskill themselves. They were paid more, recognized more, & overall had better job responsibilities than earlier monotonous & repetitive tasks.
A World Economic Forum (WEF) report predicted that, although 85 million jobs are expected to be disrupted by deep learning technologies by 2025, these same technologies are expected to create 93 million new roles, resulting in a net gain of 8 million new opening.
Just a few decades ago, the rise of the internet created similar. Despite skepticism, the technology created millions of jobs and now comprises 10% of US GDP. Today, AI is poised to create even greater growth in the US and global economies. Sixty-five percent of CEOs believe AI will have a larger impact than the internet, according to PwC’s Annual Global CEO Survey.
I believe that while the Fourth Industrial Revolution technologies driven by AI will continue to fundamentally change the world and the way we work and live, AI may not lead to massive unemployment.
Instead, AI technology will create more jobs than it automates.
Software testing is long way to go. Automation has proved to be an acceleration to Software Testing overall.
There was never a replacement by Automation even with AI / ML flavor in it for end to end system flows.
It still requires human intervention for writing scripts, updating scripts, modifying test data, take care of false positives, etc.
Exactly how ERP didn’t took jobs of silo workers and test automation didn’t replace manual testers, the rise of AI/ML won’t make manual tester irrelevant. The responsibilities would change, repeatability would be automated, there would be upskilling.
Firstly, AI relies on data to learn and make decisions. While AI can learn from historical data, it cannot always predict future scenarios or identify unanticipated errors. In contrast, humans can leverage their domain expertise to identify potential issues that AI may miss.
For instance, AI algorithms may fail to detect anomalies that are not present in training data or may misinterpret the context of the software under test. Therefore, software testing requires human expertise to complement the capabilities of AI.
***
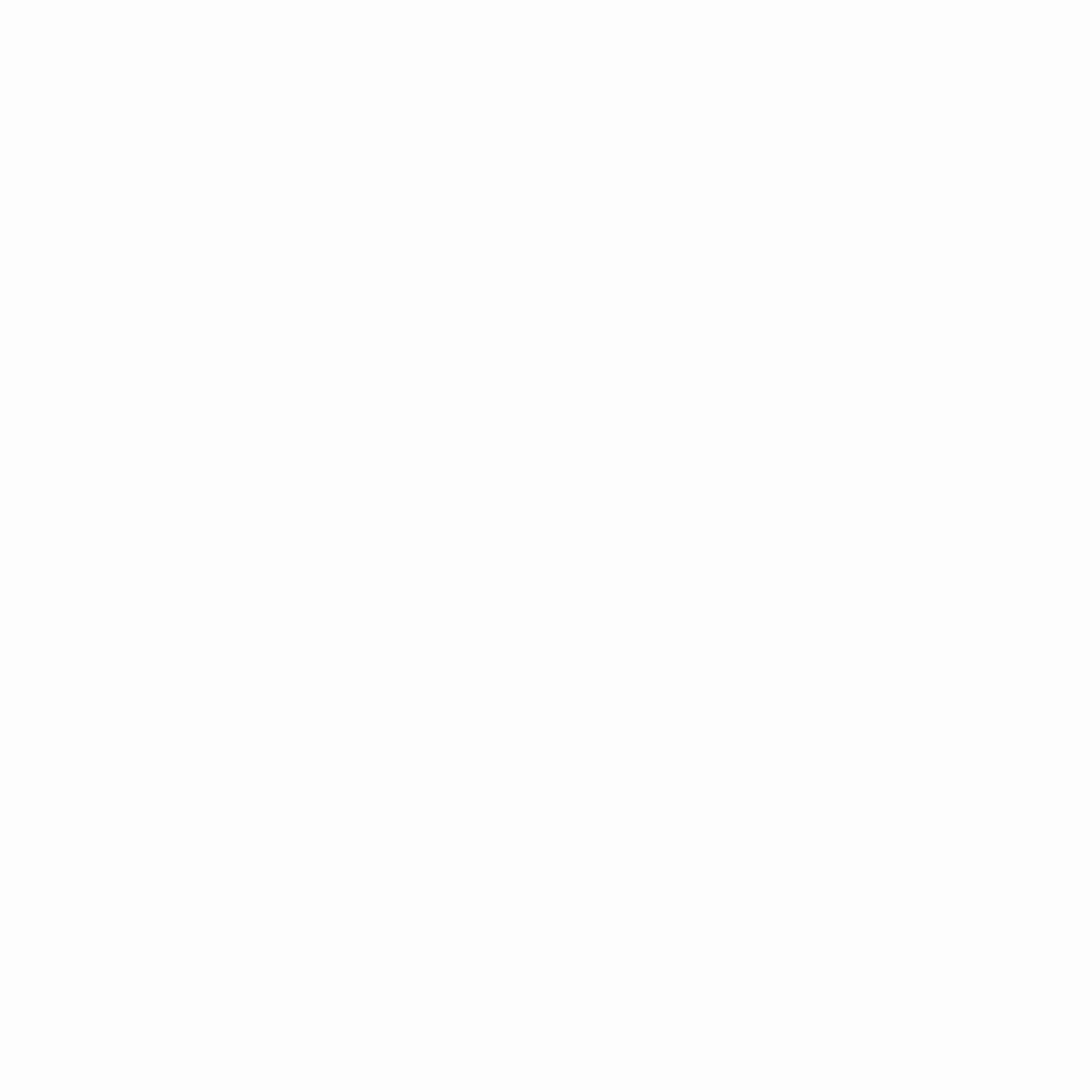
Why AI won’t make Software Testing irrelevant
● Firstly, AI relies on data to learn and make decisions. While AI can learn from historical data, it cannot always predict future scenarios or identify unanticipated errors. In contrast, humans can leverage their domain expertise to identify potential issues that AI may miss.
For instance, AI algorithms may fail to detect anomalies that are not present in training data or may misinterpret the context of the software under test. Therefore, software testing requires human expertise to complement the capabilities of AI.
● Secondly, software testing involves more than just detecting defects. It requires collaboration with developers, business analysts, and other stakeholders to understand the requirements, design test cases, and verify the functionality of software products.
Software testing also involves a degree of creativity and problem-solving skills, which AI is yet to match. Human testers can think outside the box and explore edge cases that may not be covered by automated testing.
● Thirdly, software testing involves subjective aspects such as user experience, which cannot be entirely automated. Human testers can provide feedback on the usability, accessibility, and user interface of software products, which AI may struggle to replicate.
Additionally, testing requires a comprehensive understanding of the business context, industry standards, and regulatory requirements, which can only be provided by human experts.
In software testing, the nuanced expertise of humans is essential to enhance and complement AI’s capabilities.
Conclusion
In conclusion, while AI can undoubtedly enhance software testing by automating repetitive and time -consuming tasks, it is unlikely that it will replace software testing altogether. Software testing requires human expertise, critical thinking, creativity, and collaboration, which AI cannot replicate entirely.
Therefore, rather than replacing human testers, AI can assist them in performing their jobs more efficiently and effectively, unlocking new possibilities for the future.
***
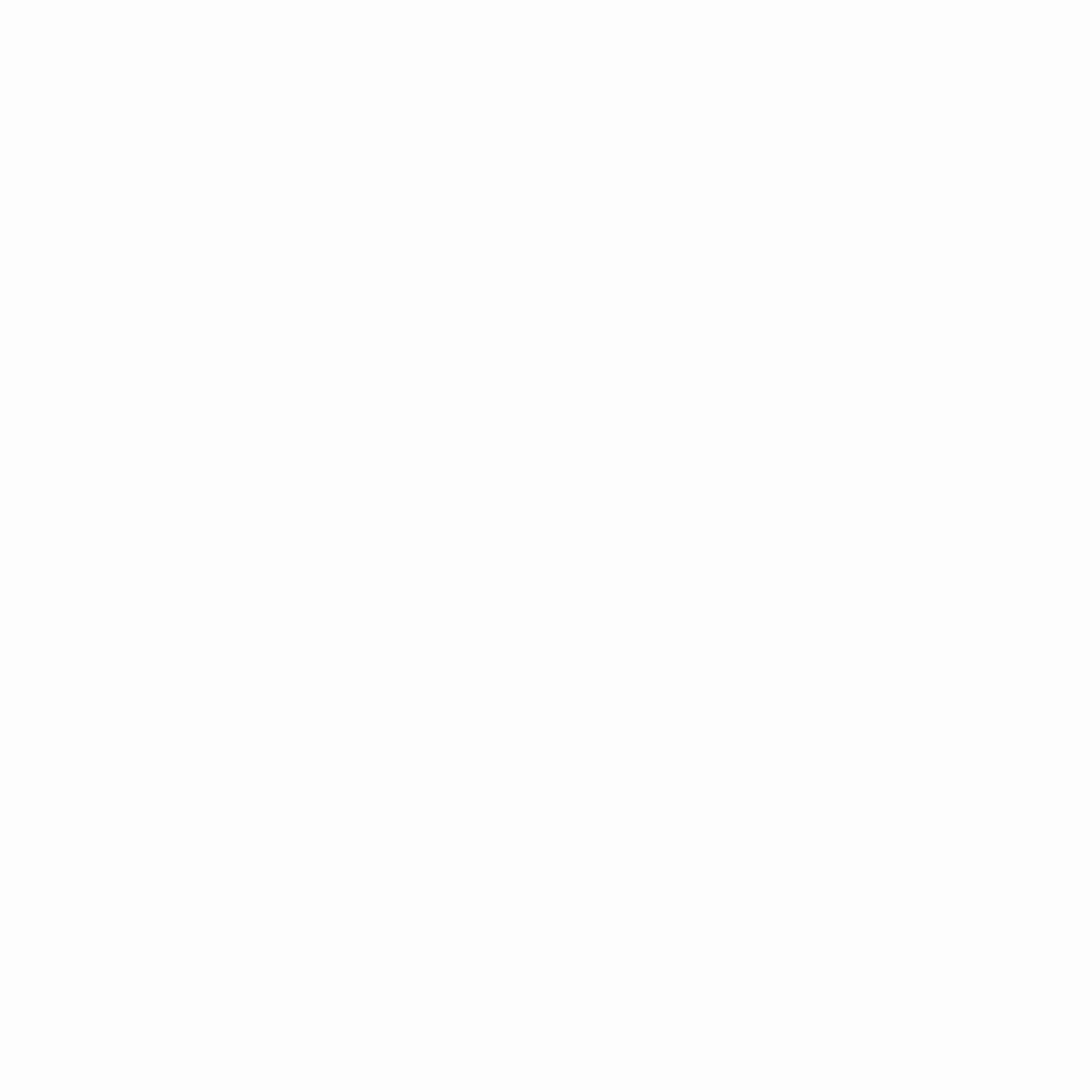
The Author
Rajat Taheem is the Delivery Manager – Verification & Validation at L&T Technology Services Limited.
Source: L&T Technology Services Limited
copyright@L&T Technology Services Limited
Disclaimer: Views are personal.